
- This event has passed.
Excess closure in a multilayer population-scale social network
21 October 2022 , 11:45 – 12:00 CEST
Lecture by Eszter Bokànyi at the Conference on Complex Systems
Recent studies on large-scale social networks successfully utilise the growing abundance of digital data sources such as online social networks or mobile communication datasets to uncover fundamental insights on human interaction [1, 2, 3].
However, in most of these social network data sources, the sample of people that are represented by the nodes is biased, and lack of demographic data makes it hard to assess representativity. Moreover, it is often not clear what exact social relations these online or communication ties represent, thus, it is difficult to interpret findings when the goal is to derive meaningful conclusions about people’s social ties [4].
We overcome a number of these drawbacks by presenting a thorough analysis of the complete structure of a 17M node population-scale social network of the Netherlands containing roughly 1.6B edges. This network is derived from highly curated official data sources of the country’s national statistics institute and includes every registered resident in 2018. The edges cover several social relationships: family, household, work, school, and neighbor. We model each of these edge types as a layer of a node-aligned multilayer network.
In addition, we have rich individual-level demographic and socio-economic attributes on the nodes (people) available. We consider the network to be a representation of the social opportunity structure in the Netherlands.
Here, we present the first results that show how this population-scale social network is markedly different from many of the large-scale social networks we typically study and reflect on the consequences for computational social science. Below, we in particular do so by revisiting the well-known concept of closure.
Closure is important because individuals have very different resource structures encoded into their social relationships throughout their lives or across demographic groups, which affects their access to opportunities and information [5]. However, if we choose to measure closure in a complete population scale social network through traditional local clustering coefficient on the separate layers, we would get values close to 1. By unioning edges from all layers, despite the average local clustering coefficient low- ering to 0.40, it is still unable to resolve potential overlaps or bridges between edges from different layers in people’s egonetworks.
To overcome this problem, we propose a normalized clustering coefficient that we call excess closure, that fully exploits the multilayer structure of the networks, and captures the fraction of triangles in people’s social circles that span across multiple types of relationships.
Figure 1 shows how degree and excess closure change with age (a demographic attribute) in the population. Young children have low degrees and very high excess closure since they are only part of family, neighborhood, and household structures. Subsequent levels of education paired with working opportunities come with both an increasing median degree, and decreasing excess closure, reaching its minimum Fig. 1. Median degree (red) and median excess closure (blue) in ego networks of people of a certain age. Shaded areas are the 25th and 75th percentiles for each age year. around the university age. Working years are characterized by a slight increase in closure, and gradually decreasing degree, giving place to low degrees and increased closure in retirement years.
Our new normalized multilayer clustering coefficient measure excess closure helps to analyse complete large-scale social networks. The measure captures overlap and bridging between edges of different types in the egonetwork of an individual. We find that excess closure varies across demographic groups as well as throughout people’s lives and it gives a more finegrained understanding of closure in multi-layer population-scale social network data. Our results show a sharp transition from closed to open network structures as young adults engage in higher levels of education, and a reverse process as people retire. These measurements are first steps in building both methods and universal insights on the rich network structure of highly curated population-level network datasets.
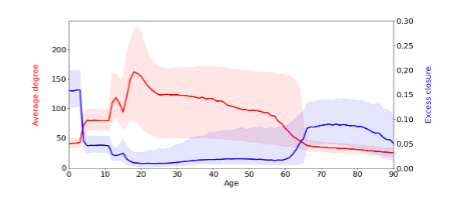
References
[1] N. Eagle, A. S. Pentland, and D. Lazer. “Inferring Friendship Network Structure by Using Mobile Phone Data.” In: Proceedings of the National Academy of Sciences of the United States of America 106.36 (2009), pp. 15274–15278.
[2] P. S. Park, J. E. Blumenstock, and M. W. Macy. “The Strength of Long-Range Ties in Population-Scale Social Networks”. In: Science 362.6421 (2018), pp. 1410–1413.
[3] M. Bailey et al. “Social Connectedness: Measurement, Determinants, and Effects”. In: Journal of Economic Perspectives 32.3 (2018), pp. 259–280.
[4] D. Lazer et al. “Meaningful Measures of Human Society in the Twenty-First Century”. In: Nature 595.7866 (2021), pp. 189–196.
[5] G. T ́oth et al. “Inequality Is Rising Where Social Network Segregation Interacts with Urban Topology”. In: Nature Communications 12.1 (2021), p. 1143